Last week, a landmark scientific paper was published in Science magazine, showcasing how a quantum device can execute a very specific algorithm exponentially faster than any classical computer, a topic I wrote on three years ago. This was not the first paper demonstrating this particular step forward (Google did it a year prior). However, it was done using light rather than circuits, it was done with a system that has been considered a ‘dark horse’ candidate for quantum computing, and perhaps most interesting, it relies much more heavily on human labor. It also is the first landmark result in quantum computing for a China-based research group.
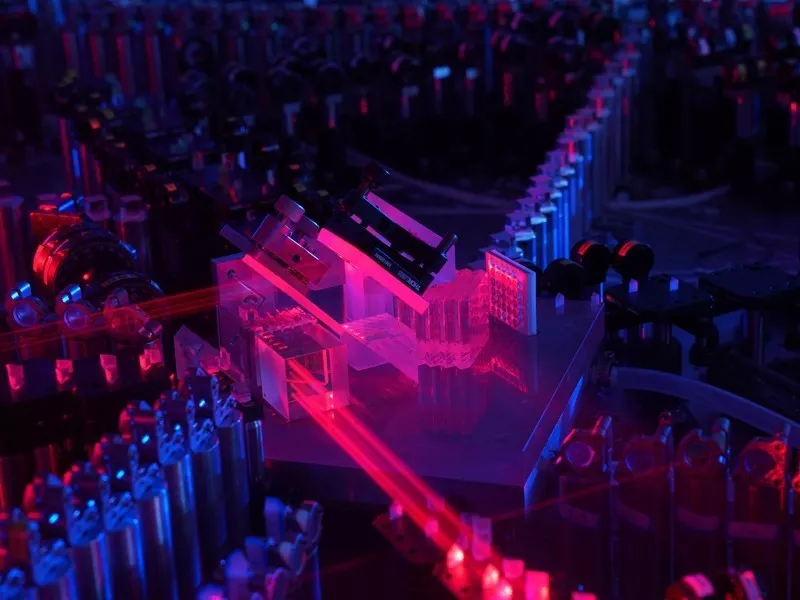
While there are a variety of intriguing ways to examine the result and its meaning, here I’d like to explore the role automation plays in physics experiments, and connect it to emerging uses of automation in other areas of our lives. With the advancing discussions around us about machine learning and autonomous vehicles, one starts to wonder how dystopian our science fiction future may become.
As a case in point, the work from Lu’s group highlights the interplay effectively: there is a tremendous amount of manual labor necessary to realize this result. If you examine the photograph from the group, you see the tremendous number of hand-aligned optical elements, each of which needs to point with micrometer precision at the right things. For me reflects a greater truth about just about every cutting edge physics experiment.
Specifically, in physics experiments (and other fields as well, but I am not an expert in automation in biology for example) the next experiment you do typically builds from your last successful experiment. Usually, you look at the apparatus and ascertain which elements you might successfully automate, so that the humans running the experiment can focus on something more important. You do this until you are no longer able to automate, then you try the experiment and hopefully succeed. I have the sense, working closely with cutting edge teams, that the limits of our knowledge are set by the rate at which we can implement this loop and understand the consequences of the increasing automation.
For example, many groups working on building quantum computers around the world on focused on how to better leverage automation. This included hardware automation, such as using nanofabrication and lithography techniques to build the complex interference systems. It also included substantial software automation in testing systems, tuning systems, and running systems, which may enable their effort to scale more rapidly than the recent Lu group results. As the CEO of Honeywell recently noted, their main value addition to the space of ion trap quantum computing is not cutting edge physics per se, but rather their expertise in control and automation of complex systems.
Takeaways for the incorporation of automation
What can we learn from the decision process for automating a part of an experimental setup? I would characterize it as driven primarily by a recognition that repetition of a job is something ideally relegated to automated systems. Usually, the decision to automate rests with the graduate student or postdoc actually doing the day-to-day automated work, and thus has a high incentive to find a more efficient use of their time. Thus the first step is having the affected community develop the initial solution.
However, the process only starts there. Once an opportunity for automation is identified, and a nascent solution prototyped, there are a variety of checks and improvements used to ensure a quality final product: namely, a reliable device for doing science. This includes other members of the lab or research team checking the work; running the system through unit tests and other means of confirming it performs within parameters and does not, for example, break the system. This takes the form of both software and hardware ‘sandboxing’: systems should have limits designated by humans are used to ensure performance the first does no harm.
As example of how this works, when I was a graduate student I wrote a feedback routine for stabilizing a large superconducting magnet in a dilution refridgerator – a system that operates a hundredth of a degree above absolute zero. Unfortunately, I had a sign error in the feedback loop, and when implemented, the magnet promptly ramped up to its maximum current. Fortunately, I had a ‘heartbeat’ code that prevent changes in the apparatus faster than the measurement system could keep up, and this prevented an accidental quench of the system and the attending venting of all the liquid helium into the room.
Finally, the system must yield results that advance the scientific goals. This means that the scientists shepherd the application and the continued evolution of the automation systems, with checks on performance and outputs. These checks are not just statistical, but also intuitive. Thus the stakeholders with expertise must continually challenge the automation systems to prove they are trending towards the intuition and the hard data expected.
These three principles form a natural core for the application of machine learning and artificial intelligence systems to problems relevant to humanity, from self-driving vehicles to new ways of discovering information and creating art. They reflect a humanist viewpoint: these systems exist to enable us, individually and as a species. Critically, they necessarily shy away from the dystopian future found in novels like Snowcrash, where advanced AI effectively hijacks the brain’s decision circuits, the science fiction future of behavioral economics and marketing on steroids. To avoid those outcomes, affected stakeholders must be part- or full-time active developers.
One part of this approach that speaks to me is the key connection between the technology, the teams that implement the technology, and the users of the technology. This is again why I look so closely at public-purpose consortia as a means of keeping these communities connected and growing in concert, together.
To summarize:
-
Community-driven automation puts humans at the key decision points for the creation of a new system
-
Sandboxing, including both software limits and engineering controls (interlocks that protect us from our own stupidity and from the ‘unknown’ unknowns), comes at the beginning, middle, and end of the projects.
-
Automated systems application and performance require stewardship, with experts and stakeholders continually challenging their outcomes and expectations and testing them against both data and intuition.
-
Communities impacted by automation should be part of the development cycle, including in their education and in their deployment.
These steps we already recognize as essential for advancing the forefront of human knowledge. I have a great hope that they can also be helpful in enabling humanity to use our time more effectively while not sacrificing the essential elements of our society, culture, and life.
Taylor, Jake. “Learning from the automation of physics experiments .” December 16, 2020